🎉 Get Started for Free! Sign up today and activate your Free Plan—no credit card required!
🚀 Launching Private Beta for Startups: Get in touch!
✨ Schedule a Demo Today and Discover How Autonmis Can Empower Your Workflow!
🎉 Get Started for Free! Sign up today and activate your Free Plan—no credit card required!
🚀 Launching Private Beta for Startups: Get in touch!
✨ Schedule a Demo Today and Discover How Autonmis Can Empower Your Workflow!
🎉 Get Started for Free! Sign up today and activate your Free Plan—no credit card required!
🚀 Launching Private Beta for Startups: Get in touch!
✨ Schedule a Demo Today and Discover How Autonmis Can Empower Your Workflow!
🎉 Get Started for Free! Sign up today and activate your Free Plan—no credit card required!
🚀 Launching Private Beta for Startups: Get in touch!
✨ Schedule a Demo Today and Discover How Autonmis Can Empower Your Workflow!
11/12/2024
Time Series Analysis: Understanding Stationarity and Its Importance
Master the fundamentals of time series analysis and learn why stationarity is crucial for accurate forecasting and analysis. A comprehensive guide for data professionals working with temporal data.
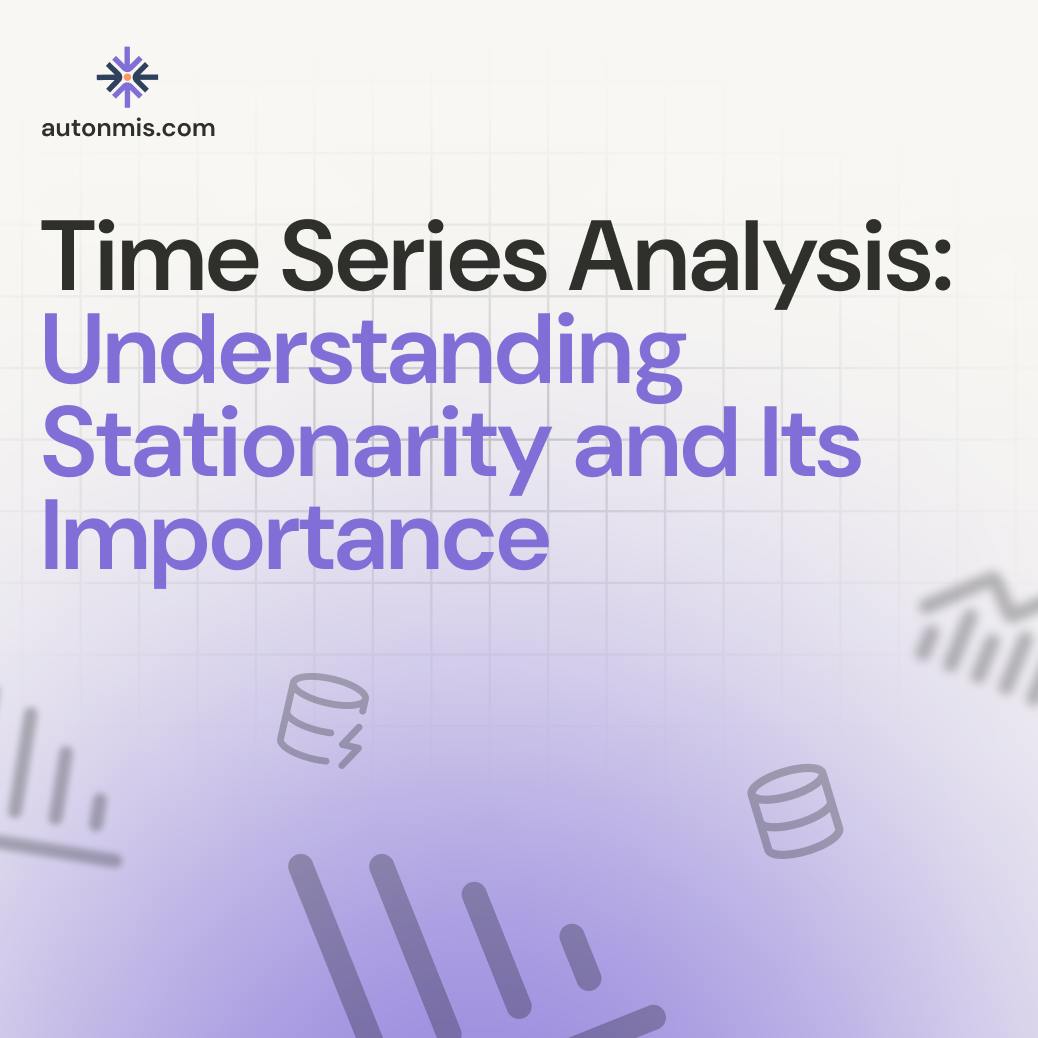
Time series analysis is fundamental to understanding and predicting patterns in sequential data. Whether you're analyzing financial markets, customer behavior, or operational metrics, understanding stationarity is crucial for accurate analysis and forecasting. This comprehensive guide will explore the concept of stationarity and its practical applications in time series analysis.
Understanding Time Series Analysis
What is Time Series Data?
Time series data consists of observations collected sequentially over time. Common examples include:
- Stock prices
- Sales figures
- Temperature readings
- Website traffic
- Sensor data
Components of Time Series
- Trend: Long-term progression
- Seasonality: Regular patterns of up and down fluctuations
- Cyclical Components: Non-periodic fluctuations
- Random Variations: Unpredictable fluctuations
Understanding Stationarity
What is Stationarity?
A time series is considered stationary when its statistical properties remain constant over time:
- Constant mean
- Constant variance
- Constant autocovariance
- No systematic trends
Types of Stationarity
- Strict Stationarity All statistical properties remain constant Rare in real-world data
- Weak Stationarity Mean and variance remain constant More common in practice
Why Stationarity Matters
Importance in Analysis
- Model Reliability Most statistical models assume stationarity Non-stationary data can lead to spurious results
- Forecasting Accuracy Stationary series are more predictable Better basis for future predictions
- Pattern Recognition Easier to identify true patterns Better understanding of underlying relationships
Testing for Stationarity
Common Tests
- Visual Inspection Plotting the time series Looking for obvious trends/patterns
- Statistical Tests
- Rolling Statistics
Making Time Series Stationary
Common Transformation Methods
- Differencing
- Logarithmic Transformation
- Removing Trend and Seasonality
Real-World Applications
1. Financial Analysis
- Stock price prediction
- Risk assessment
- Portfolio optimization
2. Business Forecasting
- Sales forecasting
- Demand prediction
- Resource planning
3. Economic Analysis
- GDP growth analysis
- Inflation rate studies
- Employment trends
Modern Tools for Time Series Analysis
Modern platforms like Autonmis simplify time series analysis through:
Integrated Analysis Environment
- Combined SQL and Python capabilities
- Direct connection to time series data sources
- AI-assisted code writing for analysis
Practical Implementation
- Connect to your time series data
- Perform stationarity tests and transformations
- Create visualizations and analysis
- Share insights with team members
Best Practices for Time Series Analysis
1. Data Preparation
- Handle missing values appropriately
- Check for and remove outliers
- Ensure consistent time intervals
2. Analysis Approach
- Start with visual inspection
- Apply appropriate tests
- Document transformations
3. Model Selection
- Consider data characteristics
- Validate assumptions
- Test multiple approaches
Conclusion
Understanding stationarity is crucial for effective time series analysis. By ensuring your time series data meets stationarity requirements, you can build more reliable models and generate more accurate forecasts. Whether you're analyzing financial data, business metrics, or other temporal data, having the right tools and knowledge is essential.
For those looking to streamline their time series analysis workflow, platforms like Autonmis provide an integrated environment where you can efficiently work with time series data. From testing stationarity to implementing complex analyses, having the right tools can significantly improve your analytical capabilities.
Ready to enhance your time series analysis? Visit Autonmis to learn how our platform can help you work more effectively with temporal data.
Recommended Learning Articles
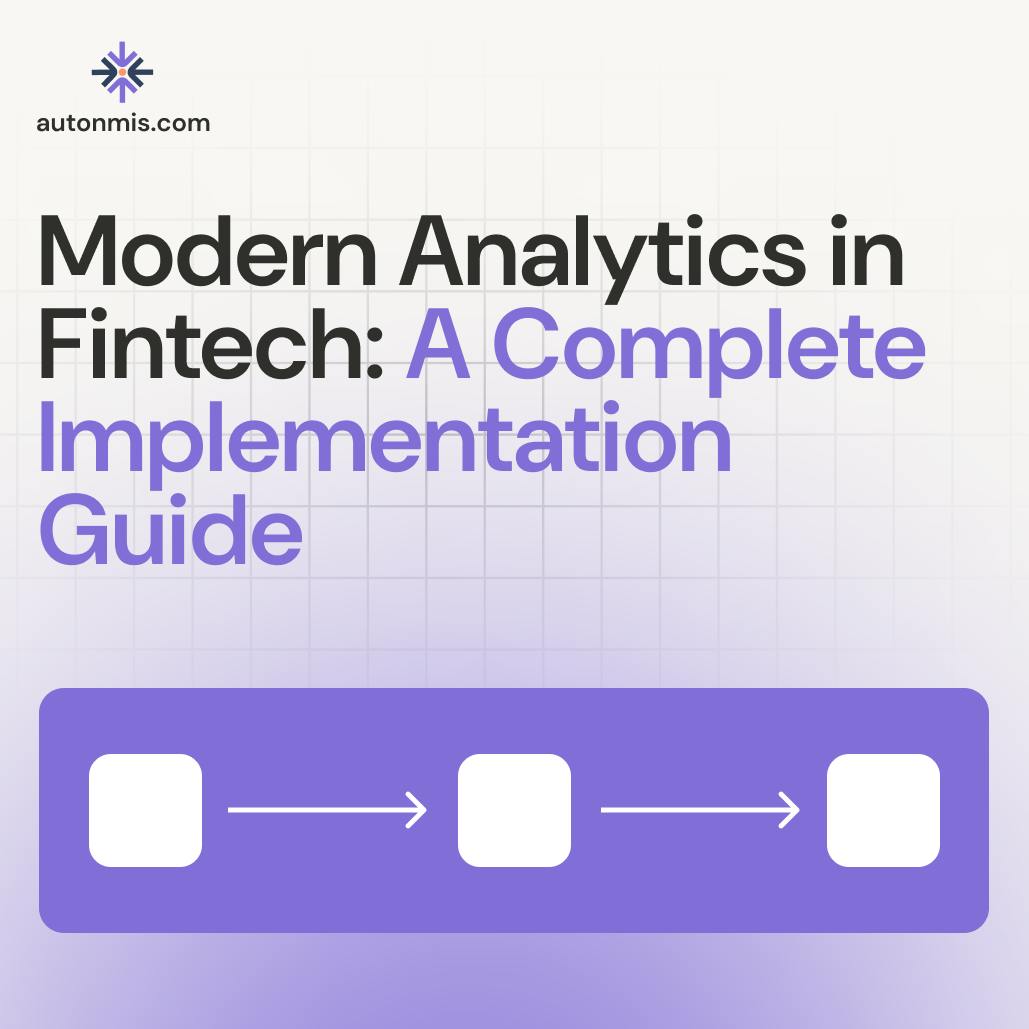
11/22/2024
Modern Data Analytics in Fintech: An Implementation Guide
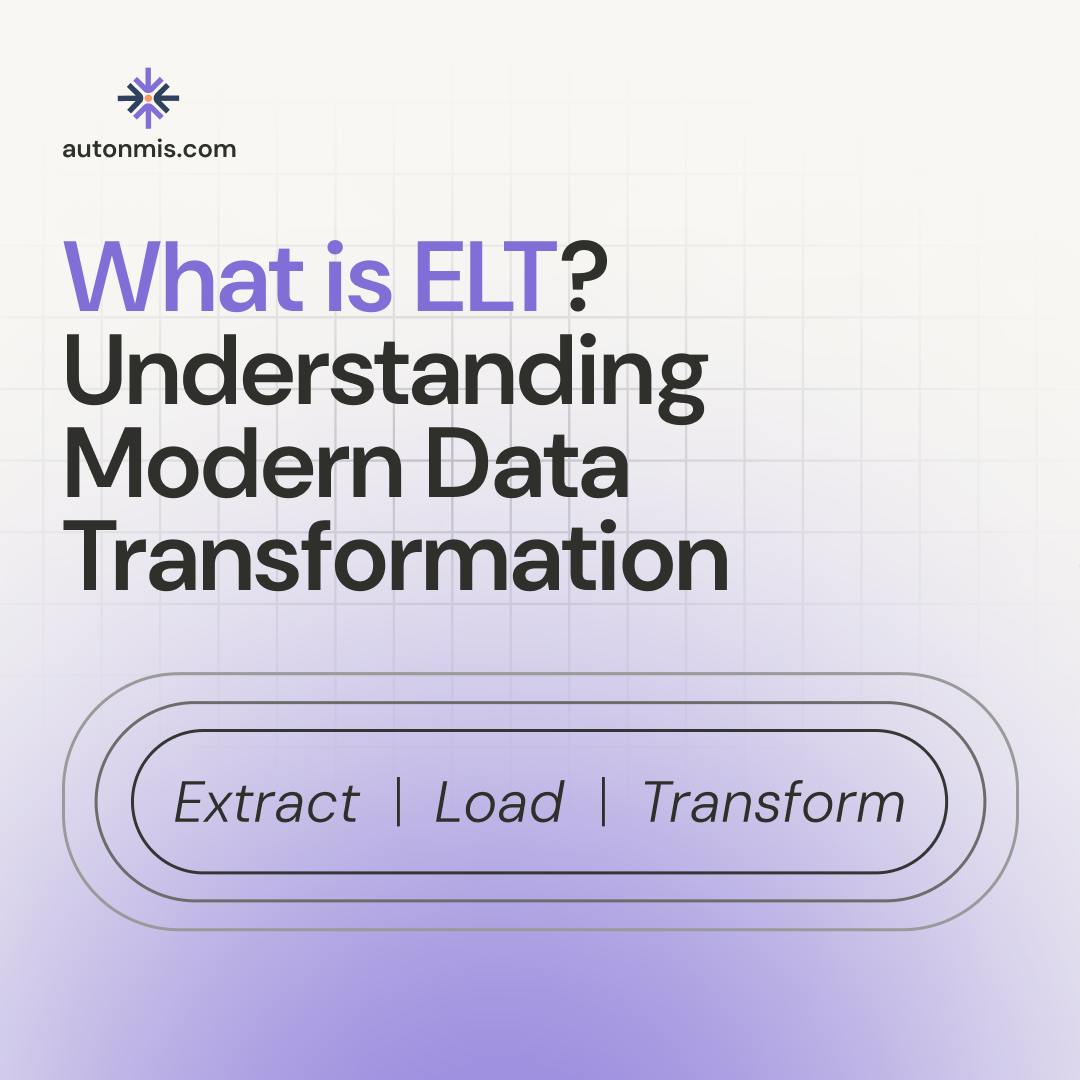
11/19/2024
What is ELT? Understanding Modern Data Transformation
Simplify your Data Work
For Enterprises, discover how scaleups and SMEs across various industries can leverage Autonmis
to bring down their TCO and effectively manage their Business Analytics stack.