🎉 Get Started for Free! Sign up today and activate your Free Plan—no credit card required!
🚀 Launching Private Beta for Startups: Get in touch!
✨ Schedule a Demo Today and Discover How Autonmis Can Empower Your Workflow!
🎉 Get Started for Free! Sign up today and activate your Free Plan—no credit card required!
🚀 Launching Private Beta for Startups: Get in touch!
✨ Schedule a Demo Today and Discover How Autonmis Can Empower Your Workflow!
🎉 Get Started for Free! Sign up today and activate your Free Plan—no credit card required!
🚀 Launching Private Beta for Startups: Get in touch!
✨ Schedule a Demo Today and Discover How Autonmis Can Empower Your Workflow!
🎉 Get Started for Free! Sign up today and activate your Free Plan—no credit card required!
🚀 Launching Private Beta for Startups: Get in touch!
✨ Schedule a Demo Today and Discover How Autonmis Can Empower Your Workflow!
9/27/2024
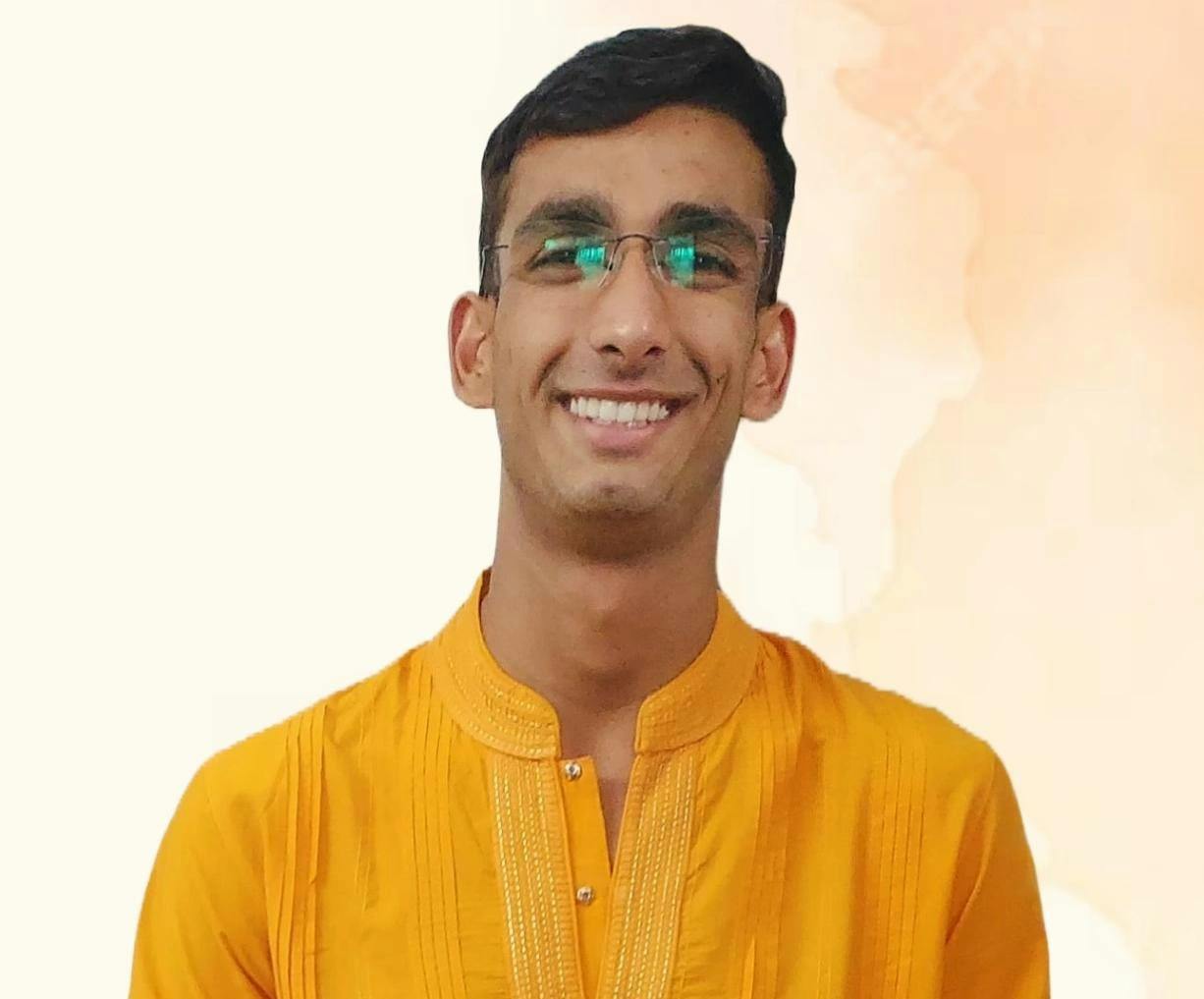
Sachin Chandra
Strategic Market Segmentation: Customer Behavior Analysis
This project analyzes customer segmentation to identify and understand distinct customer groups within a business's target market. Analyzing demographic data, purchasing ...
Introduction: Beyond the Average Customer
In the digital age of retail, the myth of the "average customer" has been shattered. Every purchase tells a story, every transaction holds a pattern, and within these patterns lie the keys to understanding the diverse tapestry of consumer behavior. This analysis delves deep into customer data to uncover these hidden patterns.
Modern retailers face a paradox: while they have more customer data than ever before, translating this data into meaningful insights remains challenging. Through sophisticated machine learning techniques, we transform raw customer data – from purchase history to campaign responses – into clear, actionable segments. Each segment represents not just a group of customers, but a unique perspective on how different consumers interact with our brand.
Our journey takes us through three critical phases: data exploration and cleaning, advanced dimensionality reduction using PCA, and finally, cluster analysis using Agglomerative Clustering. The result? Four distinct customer personas, each with their own purchasing patterns, preferences, and potential for growth.
As a first step we import the dataset into notebook environment using the convenient "Import to Notebook" option.
In this section
- Data Cleaning
- Feature Engineering
From the output we get by executing the above code in a SQL cell, we can conclude and note that:
- There are missing values in income
- Dt_Customer indicates the date a customer joined the database is not parsed as DateTime
- There are some categorical features in our data frame; as there are some features in dtype: object). So we will need to encode them into numeric forms later.
First of all, for the missing values, We are simply going to drop the rows that have missing income values.
In the next step, We are going to create a feature out of "Dt_Customer" that indicates the number of days a customer is registered in the firm's database. However, in order to keep it simple, We are taking this value relative to the most recent customer in the record.
Thus to get the values We must check the newest and oldest recorded dates.
Creating a feature ("Customer_For") of the number of days the customers started to shop in the store relative to the last recorded date
Exploratory Data Analysis
In the next bit, We will be performing the following steps to engineer some new features:
- Extract the "Age" of a customer by the "Year_Birth" indicating the birth year of the respective person.
- Create another feature "Spent" indicating the total amount spent by the customer in various categories over the span of two years.
- Create another feature "Living_With" out of "Marital_Status" to extract the living situation of couples.
- Create a feature "Children" to indicate total children in a household that is, kids and teenagers.
- To get further clarity of household, Creating feature indicating "Family_Size"
- Create a feature "Is_Parent" to indicate parenthood status
- Lastly, We will create three categories in the "Education" by simplifying its value counts.
- Dropping some of the redundant features
Now that we have some new features let's have a look at the data's stats.
The above stats show some discrepancies in mean Income and Age and max Income and age.
Do note that max-age is 128 years, As We calculated the age that would be today (i.e. 2021) and the data is old.
We must take a look at the broader view of the data. We will plot some of the selected features.
Clearly, there are a few outliers in the Income and Age features. I will be deleting the outliers in the data.
Next, let us look at the correlation amongst the features. (Excluding the categorical attributes at this point)
The data is quite clean and the new features have been included. We will proceed to the next step. That is, preprocessing the data.
DATA PREPROCESSING
In this section, We will be preprocessing the data to perform clustering operations.
The following steps are applied to preprocess the data:
- Label encoding the categorical features
- Scaling the features using the standard scaler
- Creating a subset dataframe for dimensionality reduction
All features are now numerical
All features are now scaled
DIMENSIONALITY REDUCTION
In this problem, there are many factors on the basis of which the final classification will be done. These factors are basically attributes or features. The higher the number of features, the harder it is to work with it. Many of these features are correlated, and hence redundant. This is why I will be performing dimensionality reduction on the selected features before putting them through a classifier. Dimensionality reduction is the process of reducing the number of random variables under consideration, by obtaining a set of principal variables.
Principal component analysis (PCA)Â is a technique for reducing the dimensionality of such datasets, increasing interpretability but at the same time minimizing information loss.
Steps in this section:
- Dimensionality reduction with PCA
- Plotting the reduced dataframe
Dimensionality reduction with PCA
For this project, We will be reducing the dimensions to 3.
CLUSTERING
Now that We have reduced the attributes to three dimensions, We will be performing clustering via Agglomerative clustering. Agglomerative clustering is a hierarchical clustering method. It involves merging examples until the desired number of clusters is achieved.
Steps involved in the Clustering
- Elbow Method to determine the number of clusters to be formed
- Clustering via Agglomerative Clustering
- Examining the clusters formed via scatter plot
The above cell indicates that four will be an optimal number of clusters for this data. Next, we will be fitting the Agglomerative Clustering Model to get the final clusters.
To examine the clusters formed let's have a look at the 3-D distribution of the clusters.
EVALUATING MODELS
Since this is an unsupervised clustering. We do not have a tagged feature to evaluate or score our model. The purpose of this section is to study the patterns in the clusters formed and determine the nature of the clusters' patterns.
For that, we will be having a look at the data in light of clusters via exploratory data analysis and drawing conclusions.
Firstly, let us have a look at the group distribution of clustering
The clusters are fairly distributed
Income vs spending plot shows the clusters pattern
- group 0: high spending & average income
- group 1: high spending & high income
- group 2: low spending & low income
- group 3: high spending & low income
Next, We will be looking at the detailed distribution of clusters as per the various products in the data. Namely: Wines, Fruits, Meat, Fish, Sweets and Gold
From the above plot, it can be clearly seen that cluster 1 is our biggest set of customers closely followed by cluster 0. We can explore what each cluster is spending on for the targeted marketing strategies.
Let us next explore how did our campaigns do in the past.
There has not been an overwhelming response to the campaigns so far. Very few participants overall. Moreover, no one part take in all 5 of them. Perhaps better-targeted and well-planned campaigns are required to boost sales.
Unlike campaigns, the deals offered did well. It has best outcome with cluster 0 and cluster 3. However, our star customers cluster 1 are not much into the deals. Nothing seems to attract cluster 2 overwhelmingly
PROFILING
Now that we have formed the clusters and looked at their purchasing habits. Let us see who all are there in these clusters. For that, we will be profiling the clusters formed and come to a conclusion about who is our star customer and who needs more attention from the retail store's marketing team.
To decide that I will be plotting some of the features that are indicative of the customer's personal traits in light of the cluster they are in. On the basis of the outcomes, I will be arriving at the conclusions.
Profiling The Clusters
About Cluster Number: 0
• Are definitely a parent
• At the max have 4 members in the family and at least 2
• Single parents are a subset of this group
• Most have a teenager at home
• Relatively older
About Cluster Number: 1
• Are definitely not a parent
• At the max are only 2 members in the family
• A slight majority of couples over single people
• Span all ages
• A high-income group
About Cluster Number: 2
• The majority of these people are parents
• At the max are 3 members in the family
• They majorly have one kid (and not teenagers, typically)
• Relatively younger
About Cluster Number: 3
• They are definitely a parent
• At the max are 5 members in the family and at least 2
• Majority of them have a teenager at home
• Relatively older
• A lower-income group
Conclusion: From Customer Segmentation Insights to Action
Our analysis reveals a nuanced picture of customer behavior that challenges conventional marketing wisdom. The four distinct clusters we've identified – from high-spending parents to deal-seeking young families – each represent unique opportunities and challenges for targeted marketing strategies.
Key Discoveries:
- The Premium Segment (Cluster 1): High-income, typically childless customers who respond well to luxury products but show limited interest in deals
- Family Value Seekers (Cluster 0): Middle-income parents who actively engage with deals and promotions
- Budget Conscious (Cluster 2): Younger families with modest spending patterns
- Aspirational Buyers (Cluster 3): Lower-income families with selective high-value purchases
These insights provide a foundation for:
- Tailored marketing campaigns aligned with each segment's preferences
- Product mix optimization based on segment-specific purchasing patterns
- Improved customer engagement through personalized communication strategies
- More efficient allocation of marketing resources
The path forward is clear: transform these segments into actionable marketing strategies that speak directly to each group's unique needs and preferences. Success in modern retail isn't just about what you sell – it's about who you're selling to and how well you understand their story.
Get started with Strategic Market Segmentation: Customer Behavior Analysis
Click below to copy this free template.
Recommended Templates
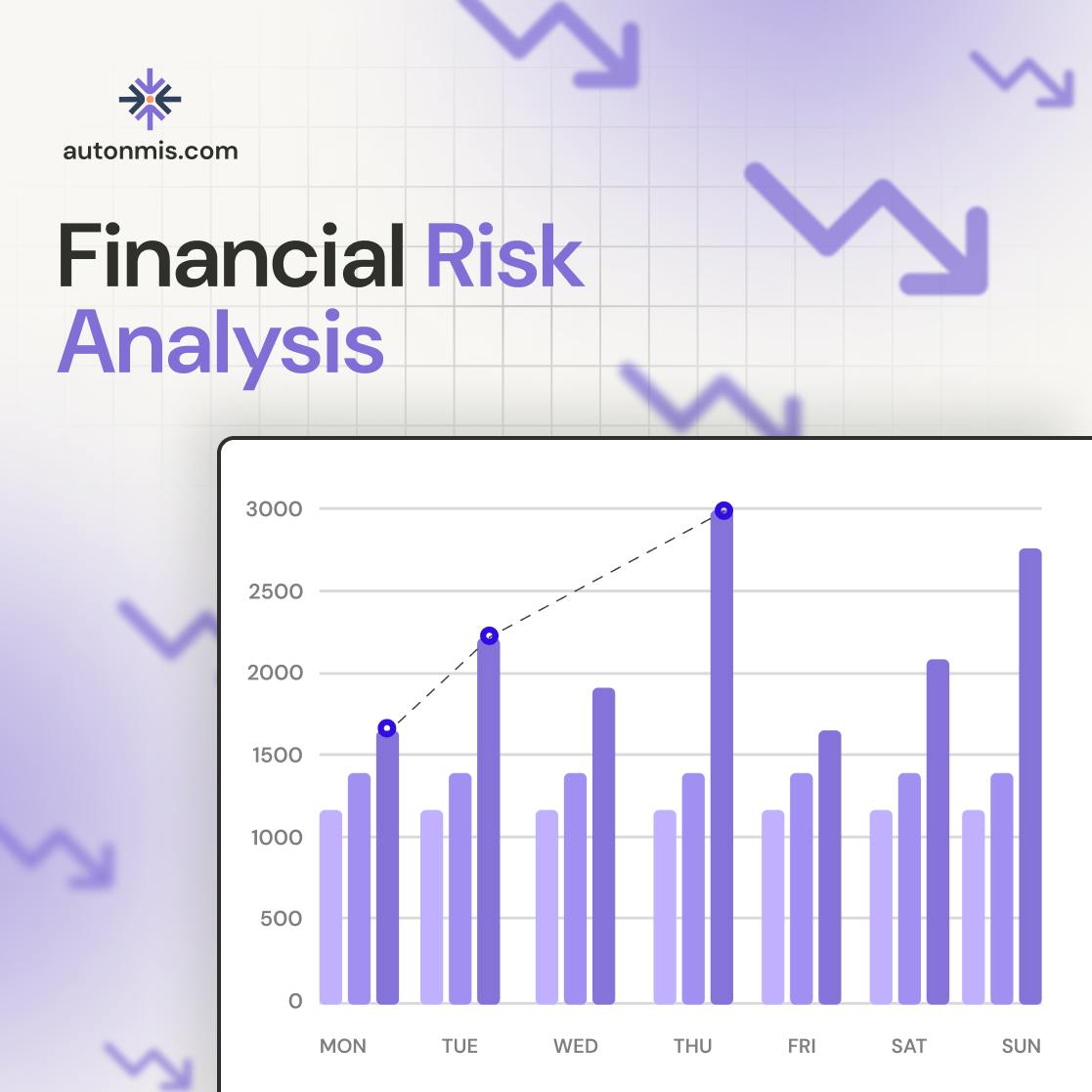
11/26/2024
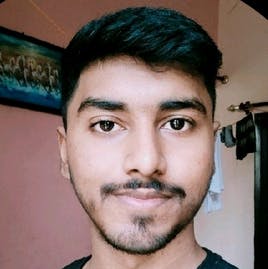
Ritik Raj
Financial Risk Analysis
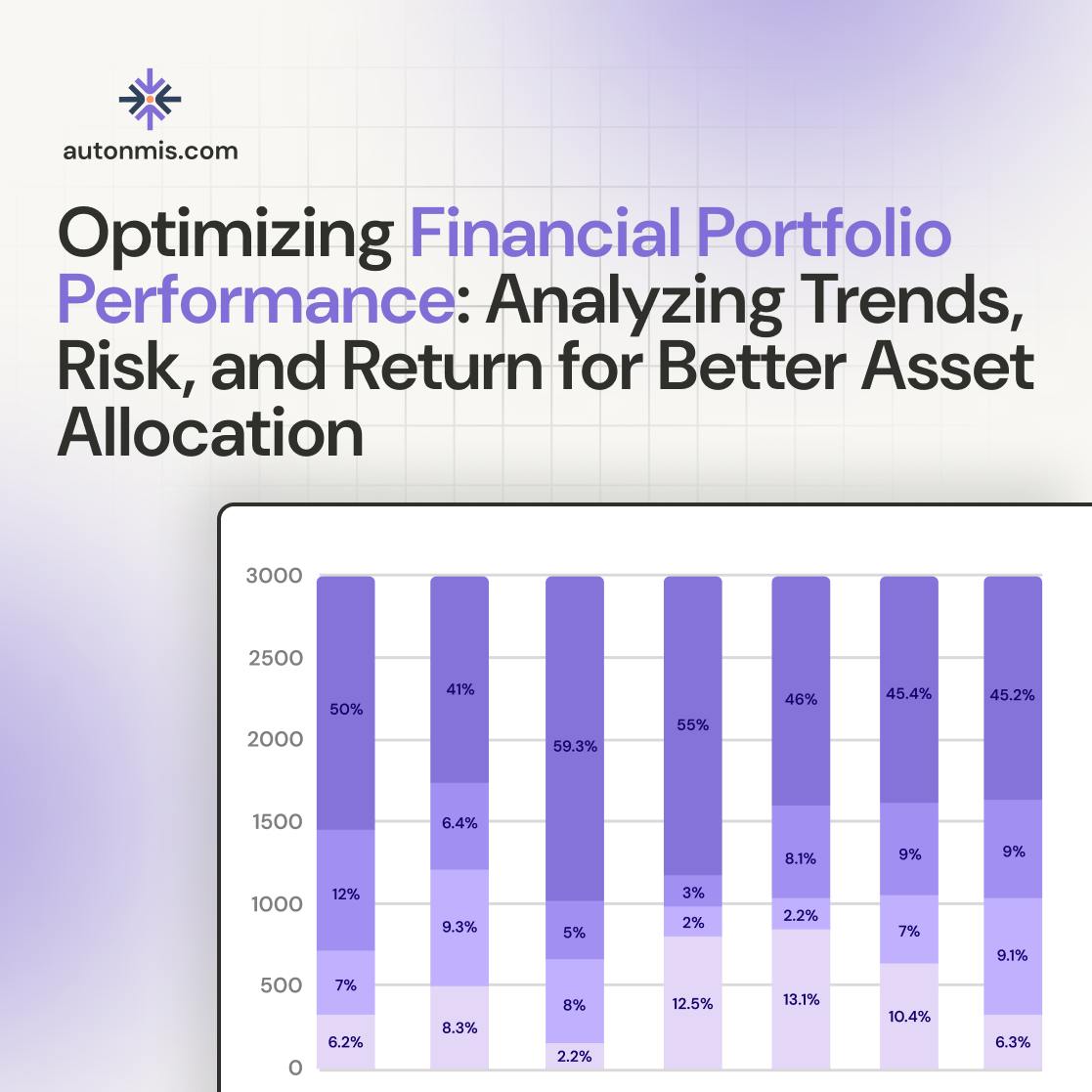
11/22/2024
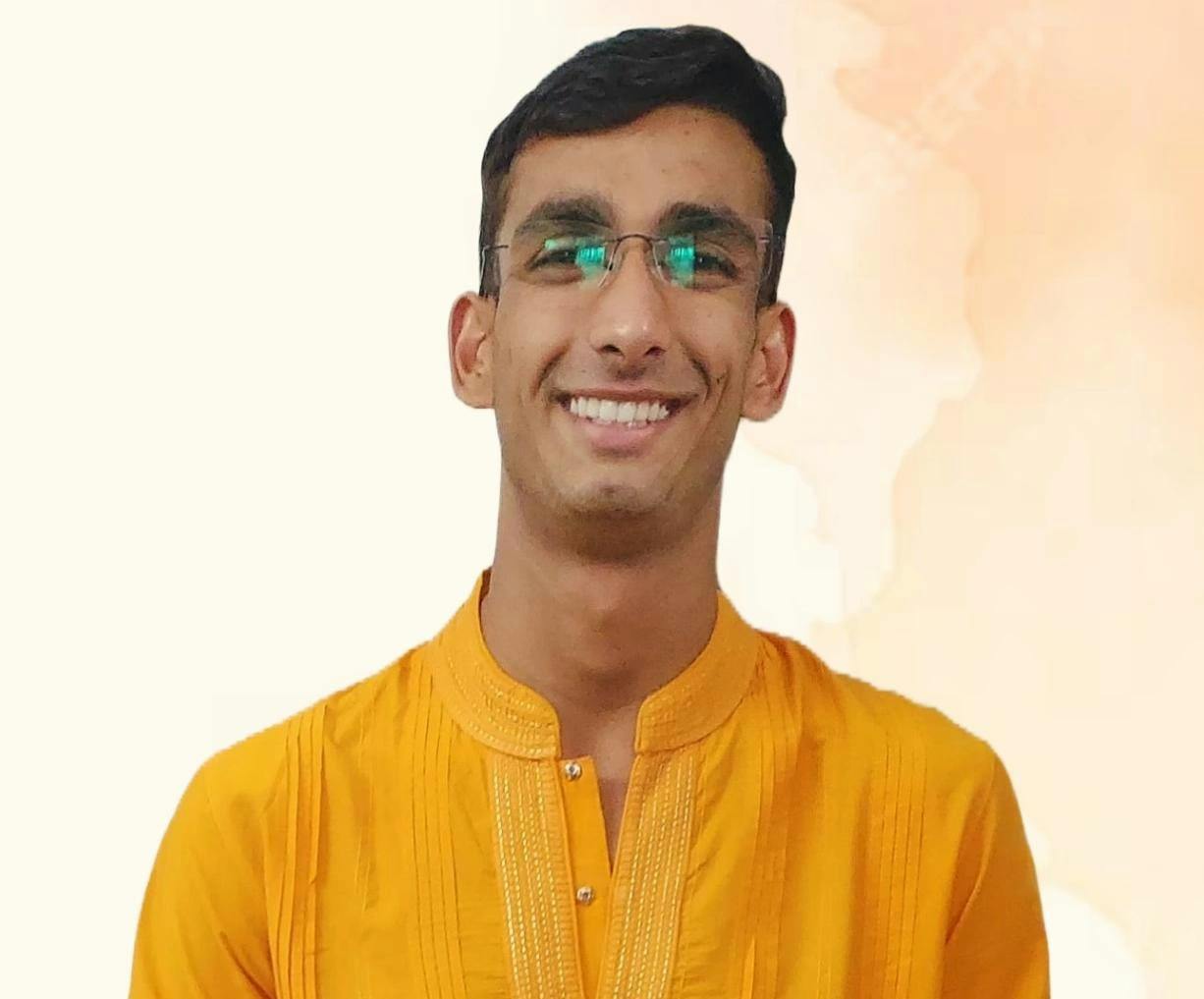
Sachin Chandra
Optimizing Financial Portfolio Performance: Analyzing Trends, Risk, and Return for Better Asset Allocation
Simplify your Data Work
For Enterprises, discover how scaleups and SMEs across various industries can leverage Autonmis
to bring down their TCO and effectively manage their Business Analytics stack.