Table of Contents
No table of contents available
From SQL to Scale: Navigating B2B SaaS Data Transformation
As organizations grow, their data transformation needs evolve from simple SQL queries to complex, multi-step processes. This journey, while necessary, often challenges teams to balance sophistication with maintainability. Let's explore how growing organizations can effectively evolve their transformation capabilities.
December 2, 2024
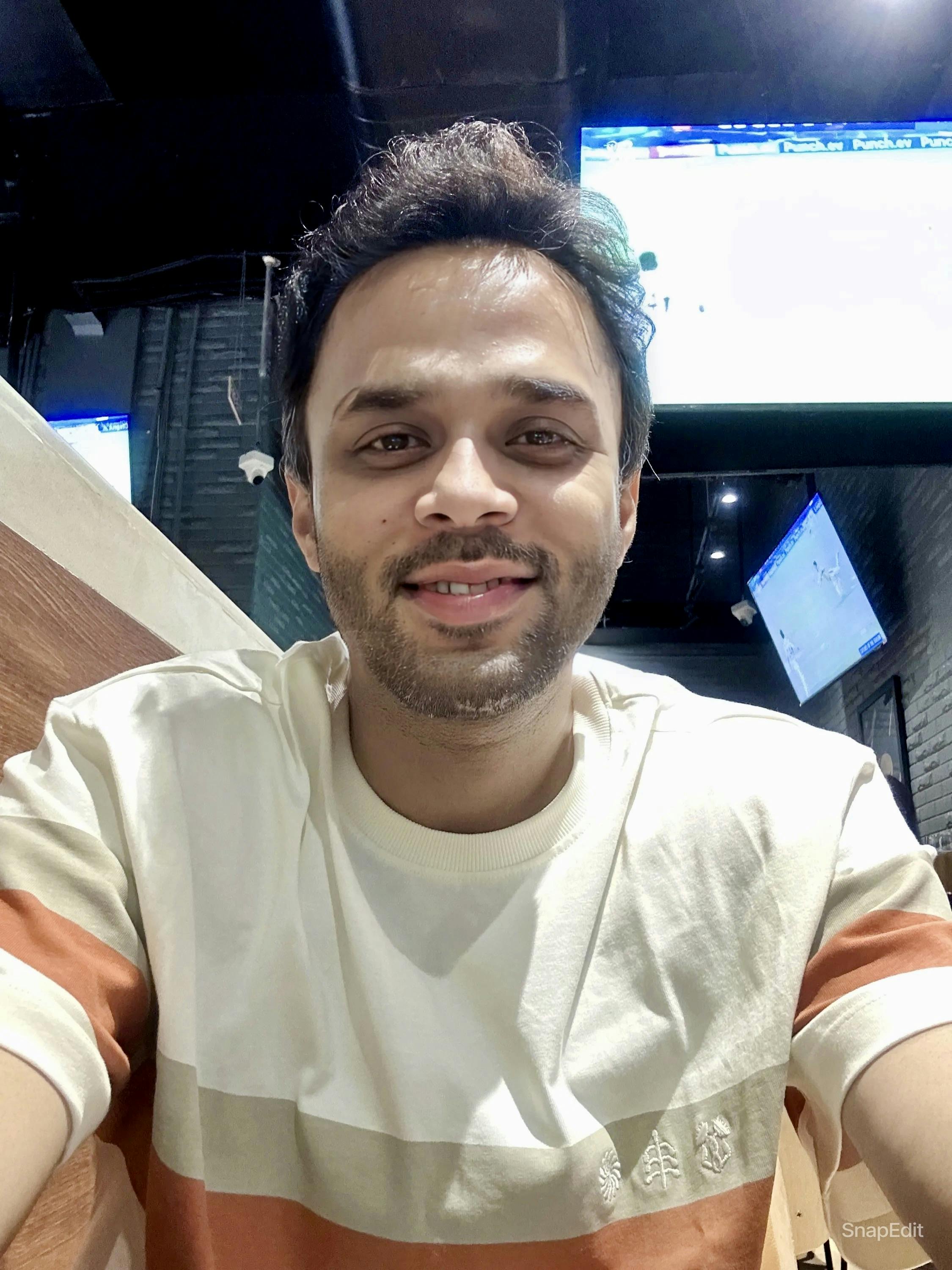
AB
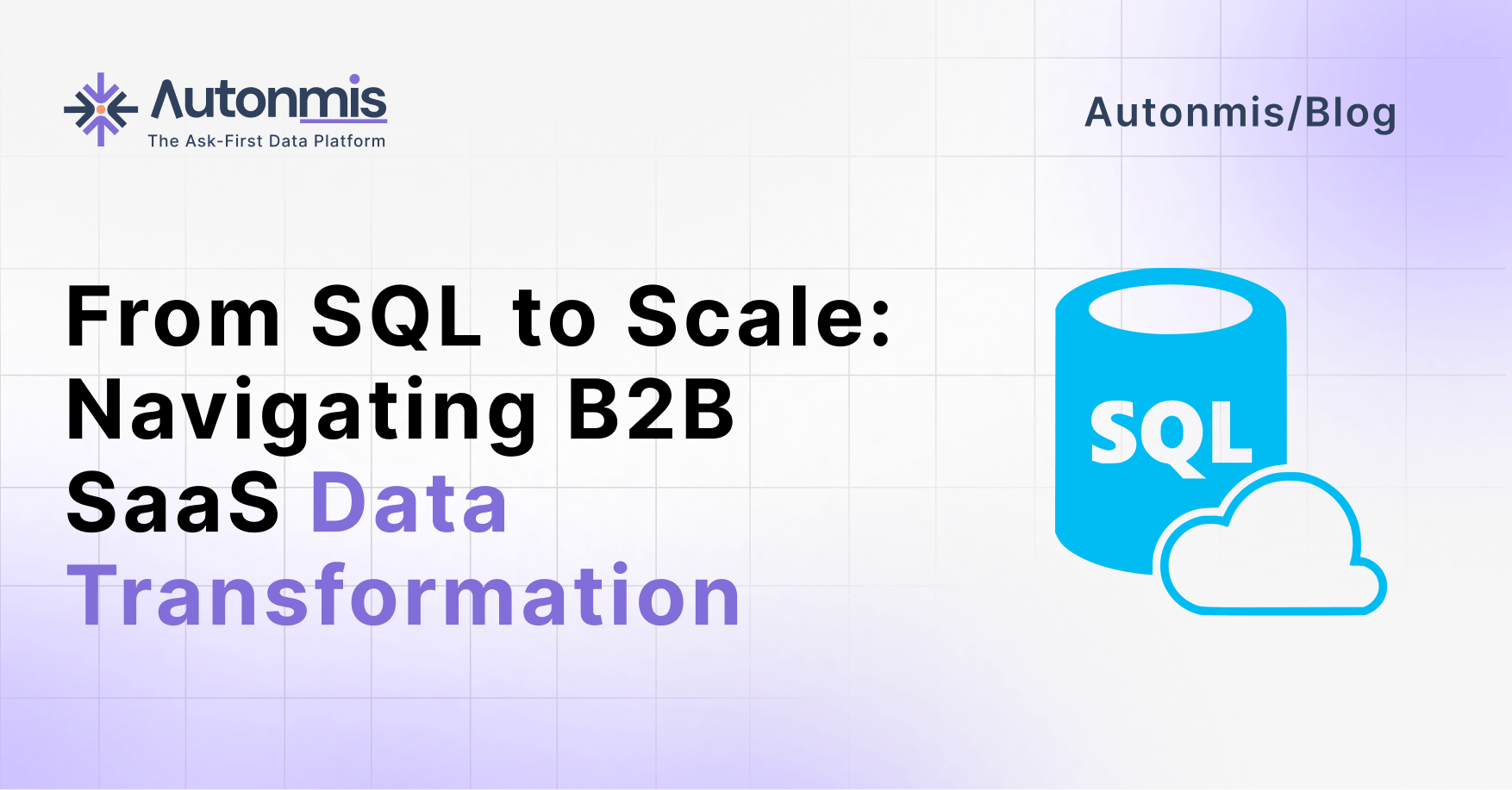
The Data Transformation Journey
Consider a typical B2B SaaS company's progression:
Initial Stage:
- Simple customer usage reports
- Basic revenue calculations
- Straightforward user metrics
Growth Stage Needs:
- Complex pricing tier analysis
- User behavior patterns
- Product usage predictions
- Customer health scoring
- Revenue attribution modeling
This evolution brings new challenges:
- Complex business logic that exceeds SQL's capabilities
- Performance issues with large-scale transformations
- Need for reusable transformation logic
- Requirements for testing and validation
Common Growth Challenges
1. The Limits of Simple SQL
Most organizations start with basic SQL transformations:
- Direct queries against production databases
- Simple joins and aggregations
- Basic filtering and grouping
Why It Breaks Down:
- Complex business logic becomes unmanageable
- Performance degrades with data volume
- Testing becomes difficult
- Reusability is limited
- Version control is challenging
2. The Maintenance Burden
As transformations grow more complex:
- SQL queries become harder to maintain
- Business logic is scattered across queries
- Changes require extensive testing
- Documentation becomes outdated
- Knowledge sharing becomes difficult
Modern Transformation Patterns
1. Modular Transformation Design
Key Concepts:
- Break complex transformations into logical units
- Create reusable transformation blocks
- Implement clear input/output contracts
- Enable easy testing and validation
Example Structure:
- Data Cleaning Layer Standardization rules Missing value handling Deduplication logic
- Business Logic Layer Customer segmentation rules Revenue attribution logic Usage pattern analysis
- Aggregation Layer Metric calculations Report generation KPI computations
2. Advanced Data Transformation Capabilities
Modern Approaches:
- Hybrid Transformations SQL for data heavy operations Python for complex logic Specialized functions for specific needs
- Intelligent Processing Automated data type handling Smart null value management Dynamic schema adaptation
- Business Logic Management Centralized rule management Versioned transformations Reusable business logic
Example of handling changing business rules and data patterns:
Example of implementing comprehensive testing and validation:
Implementation Strategies
1. Start with Clear Data Contracts
Define clear expectations for:
- Input data requirements
- Output data formats
- Quality standards
- Performance requirements
2. Build Progressive Complexity
Layer your transformation approach:
- Foundation Layer Basic data cleaning Simple transformations Essential calculations
- Business Logic Layer Complex calculations Multi-step transformations Custom business rules
- Advanced Analytics Layer Predictive features Complex aggregations Custom metrics
3. Implement Quality Controls
Essential quality measures:
- Input data validation
- Transformation logic testing
- Output data verification
- Performance monitoring
Real-World Implementation Guide
1. Assessment Phase
- Document current transformation needs
- Identify pain points
- Map future requirements
- Define success metrics
2. Foundation Building
- Implement basic transformation framework
- Set up testing environment
- Establish monitoring basics
- Create documentation structure
3. Progressive Enhancement
- Add advanced features incrementally
- Build reusable components
- Enhance monitoring capabilities
- Improve documentation
Best Practices for Growing Organizations
- Start with Standards Define naming conventions Establish coding standards Create documentation requirements Set up review processes
- Focus on Maintainability Build modular transformations Implement clear error handling Create comprehensive tests Maintain updated documentation
- Plan for Scale Design for performance Build in monitoring Plan for data growth Consider future needs
- Enable Collaboration Build shared libraries Document processes Create reusable components Establish review procedures
Modern Transformation Made Simple
Growing organizations need transformation capabilities that balance power with simplicity. A modern approach delivers:
- Low-code transformation builders with AI assistance for complex logic development
- Flexible environments that combine SQL and Python for comprehensive data processing
- Built-in testing and validation with automatic quality checks
- Smart optimization that ensures efficient resource usage while maintaining data quality
Conclusion
The evolution of data transformations is a crucial journey for growing organizations. Success comes not from adopting the most advanced solutions immediately, but from building a solid foundation that can evolve with your needs. Focus on:
- Creating maintainable transformations
- Building reusable components
- Implementing proper testing
- Enabling team collaboration
Remember: The goal is to create a transformation system that not only meets your current needs but can grow with your organization while remaining maintainable and efficient.
Ready to Scale Your Data Operations?
Start with a proven platform that combines enterprise capabilities with startup agility. Schedule a demo to see how Autonmis can simplify your data management.
Recommended Blogs
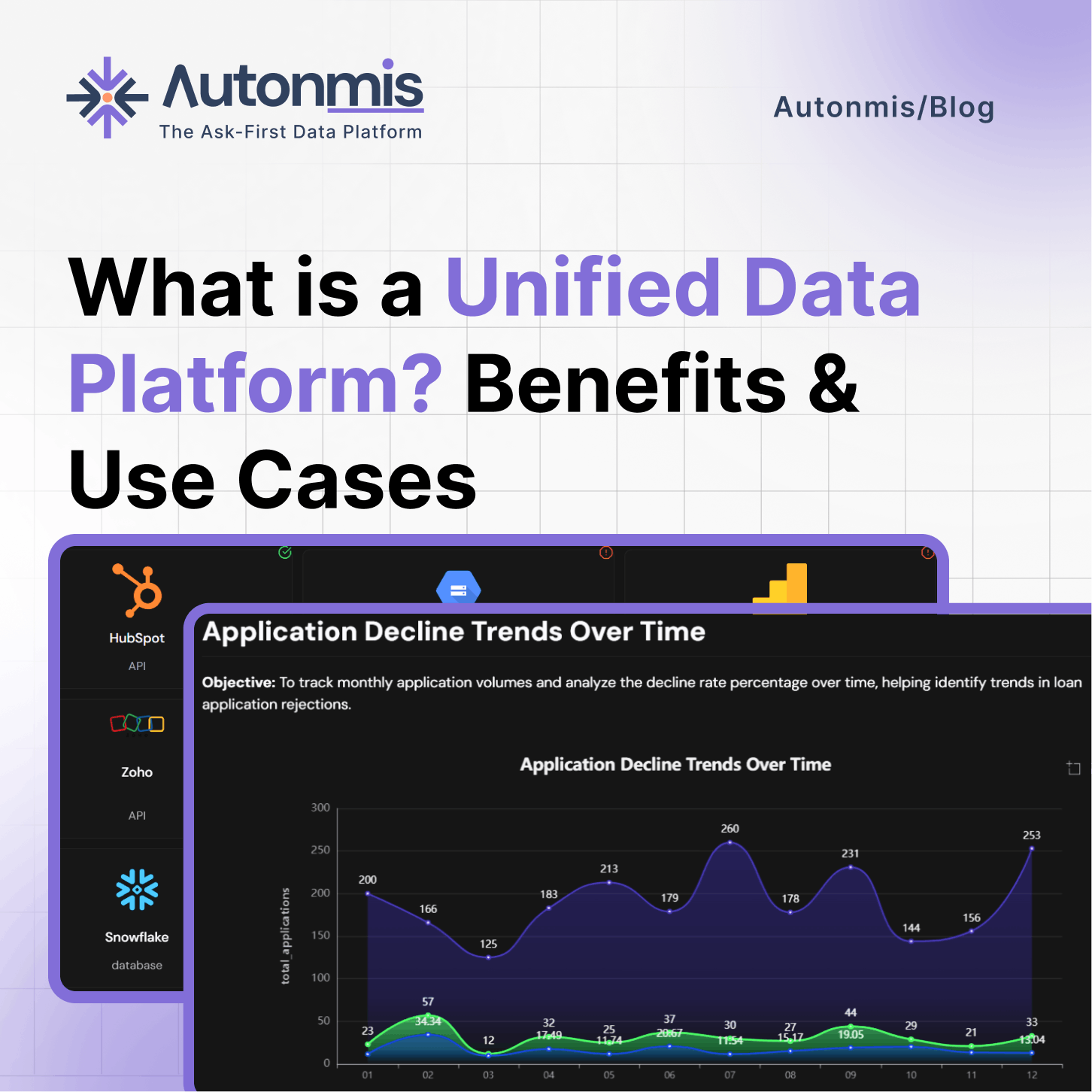
5/23/2025
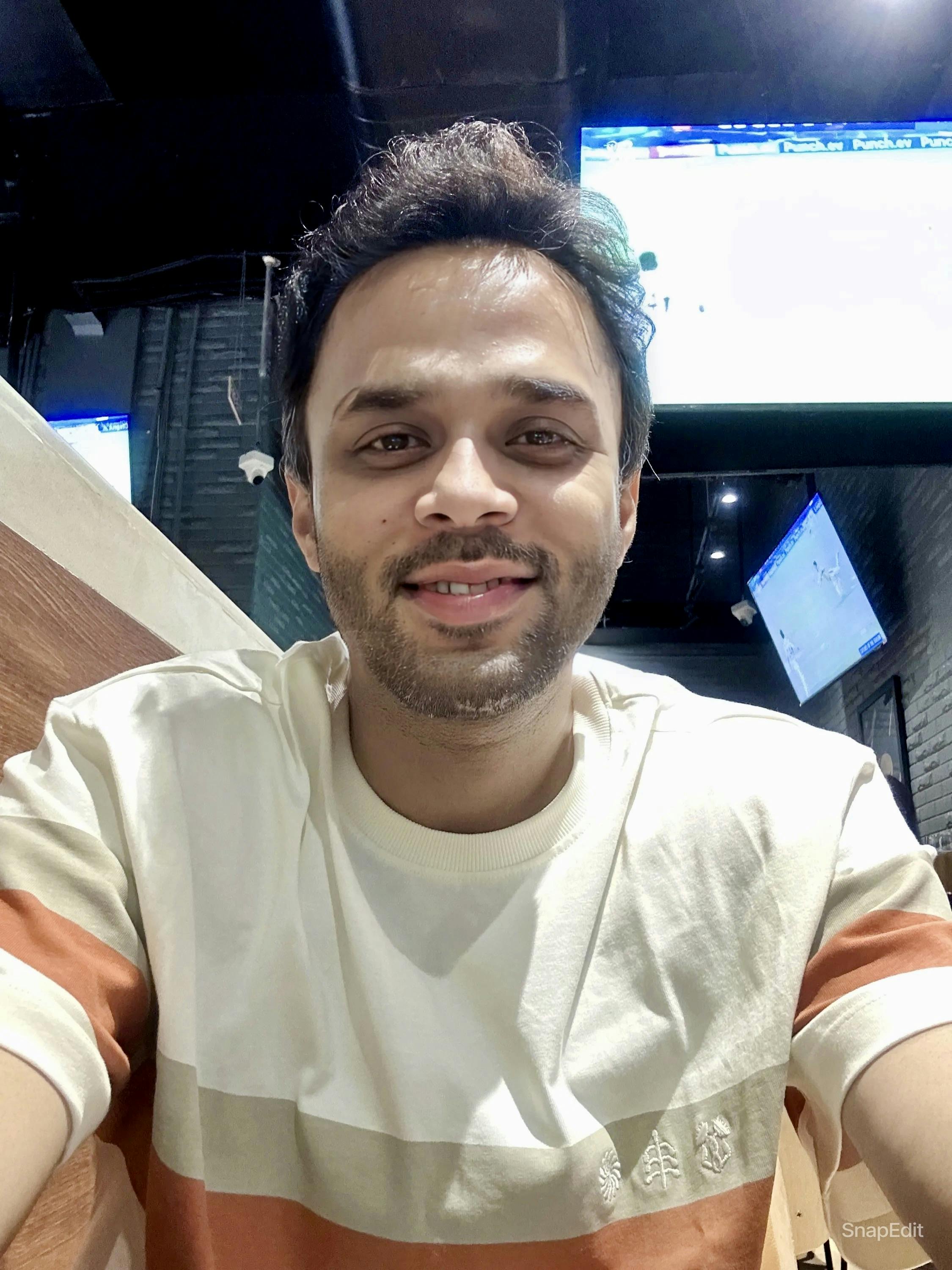
AB
What is a Unified Data Platform and Why Do Businesses Need One?
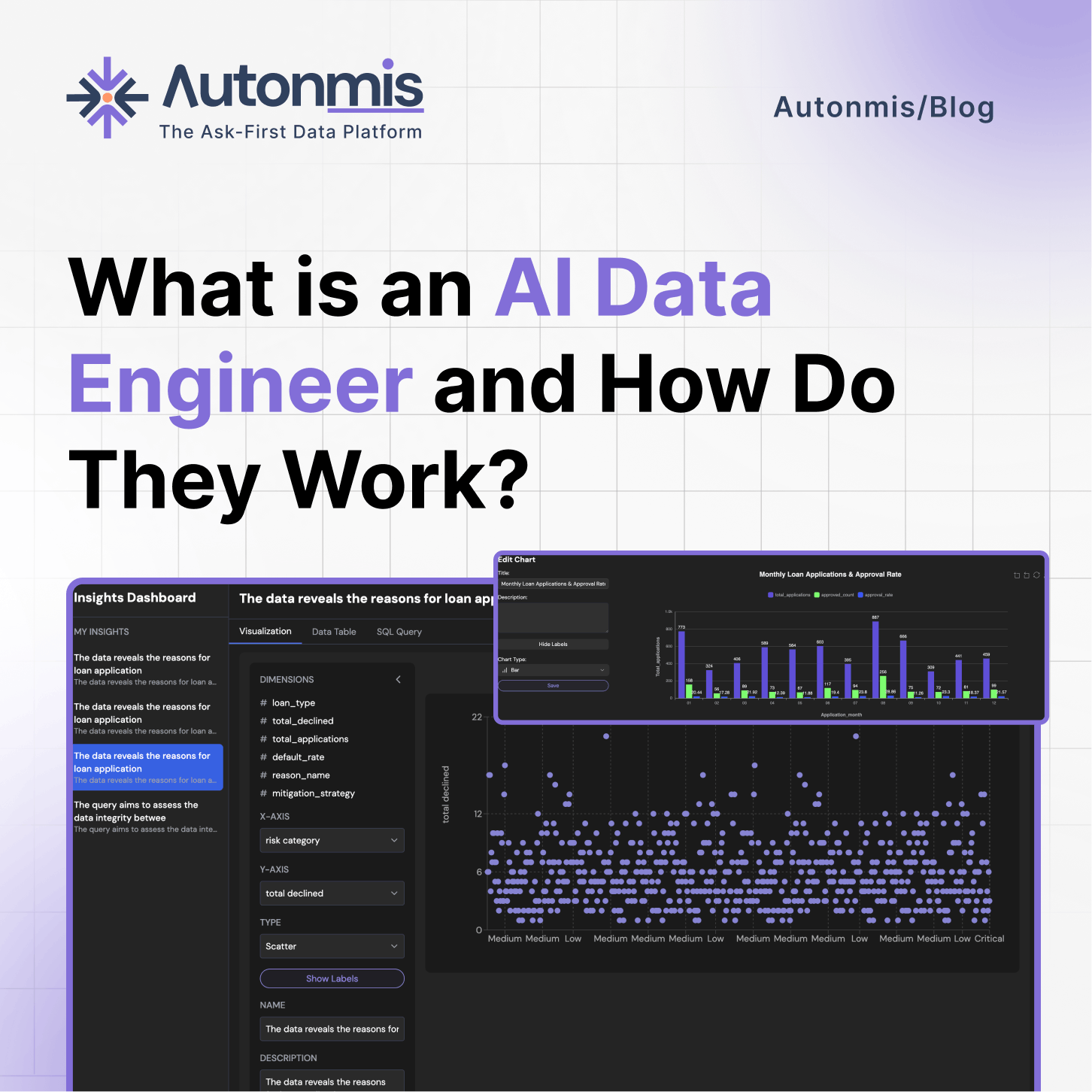
5/16/2025
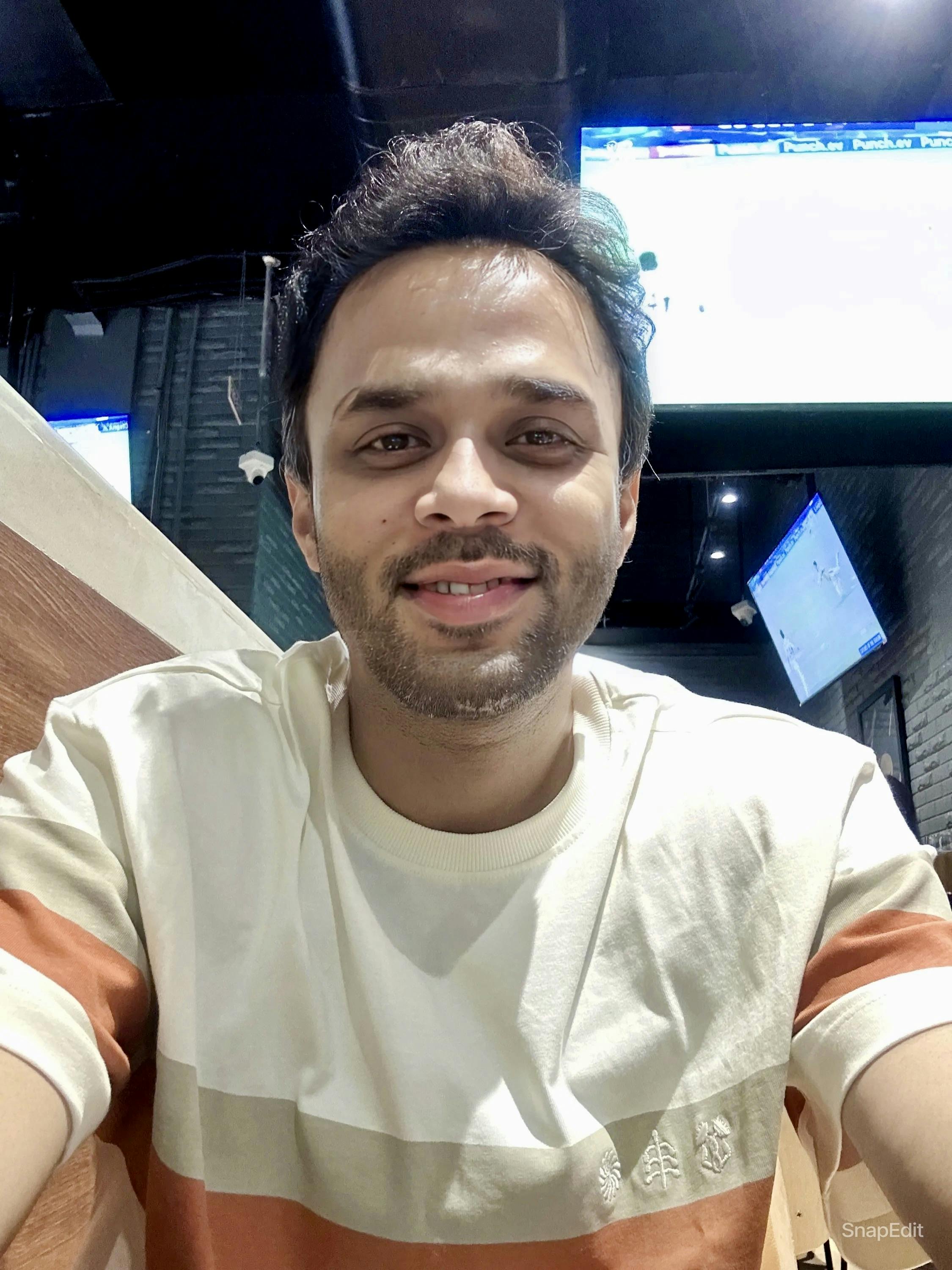
AB
What is an AI Data Engineer and How Do They Work?
Data stack that builds & runs itself
Autonmis helps scaleups and SMEs own their entire data workflow through conversation — fast, simple, and cost-effective.